
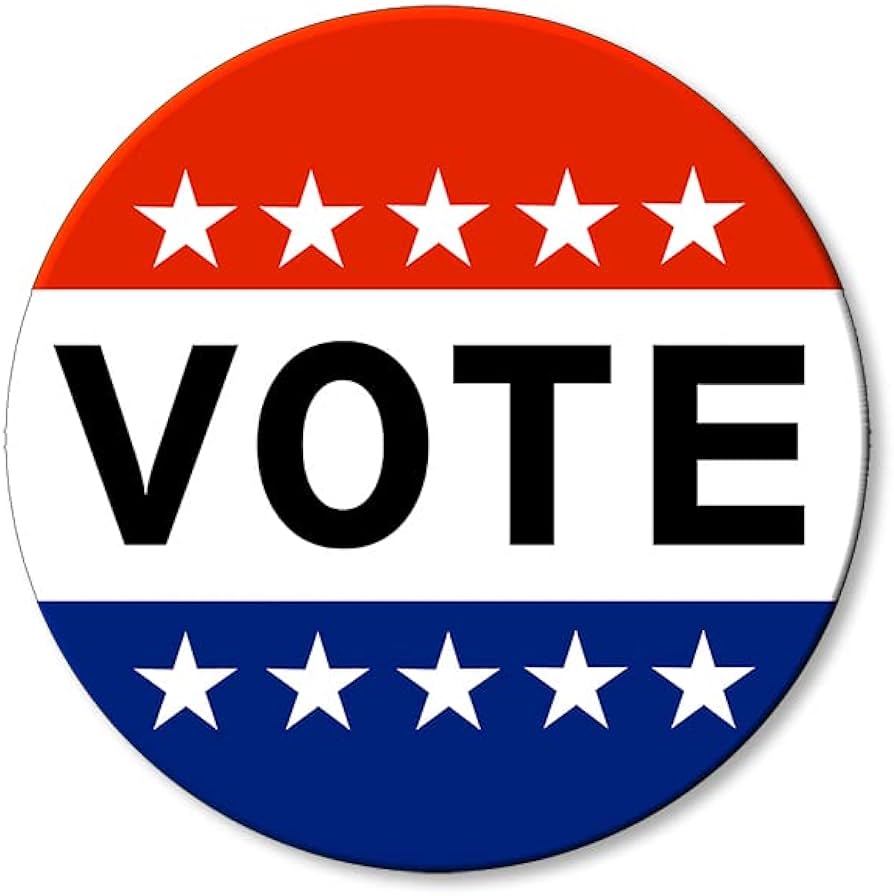
Banks hate this simple trick
Banks hate this simple trick
Blowing up? Seen conservative discussion areas? Their godking is not only one of the working class now, but he owned all the democrats and made Harris look like a fool. He’s a master troll doing 4d chess!
They built a space laser?
It’s a watch that says you have no taste.
They know their target demographic
Hah. Snake oil vendors will still sell snake oil, CEO will still be dazzled by fancy dinners and fast talking salesmen, and IT will still be tasked with keeping the crap running.
This has a lot of “I can use the bus perfectly fine for my needs, so we should outlaw cars” energy to it.
There are several systems, like firewalls , switches, routers, proprietary systems and so on that only has a manual process for updating, that can’t be easily automated.
Most phones these days use randomized MACs
https://www.guidingtech.com/what-is-mac-randomization-and-how-to-use-it-on-your-devices/
Not sure if that is for BT too, but looks like there is some support for it in the standards
https://novelbits.io/how-to-protect-the-privacy-of-your-bluetooth-low-energy-device/
https://novelbits.io/bluetooth-address-privacy-ble/
The recommendation per the Bluetooth specification is to have it change every 15 minutes (this is evident in all iOS devices).
So seems like it is implemented on some phones at least
https://www.bluetooth.com/blog/bluetooth-technology-protecting-your-privacy/
From 2015. So this seems to be a solved problem for a decade now
That’s because they don’t see the letters, but tokens instead. A token can be one letter, but is usually bigger. So what the llm sees might be something like
When seeing it like that it’s more obvious why the llm’s are struggling with it
In many cases the key exchange (kex) for symmetric ciphers are done using slower asymmetric ciphers. Many of which are vulnerable to quantum algos to various degrees.
So even when attacking AES you’d ideally do it indirectly by targeting the kex.
I generally agree with your comment, but not on this part:
parroting the responses to questions that already existed in their input.
They’re quite capable of following instructions over data where neither the instruction nor the data was anywhere in the training data.
They’re completely incapable of critical thought or even basic reasoning.
Critical thought, generally no. Basic reasoning, that they’re somewhat capable of. And chain of thought amplifies what little is there.
No, all sizes of llama 3.1 should be able to handle the same size context. The difference would be in the “smarts” of the model. Bigger models are better at reading between the lines and higher level understanding and reasoning.
Wow, that’s an old model. Great that it works for you, but have you tried some more modern ones? They’re generally considered a lot more capable at the same size
Increase context length, probably enable flash attention in ollama too. Llama3.1 support up to 128k context length, for example. That’s in tokens and a token is on average a bit under 4 letters.
Note that higher context length requires more ram and it’s slower, so you ideally want to find a sweet spot for your use and hardware. Flash attention makes this more efficient
Oh, and the model needs to have been trained at larger contexts, otherwise it tends to handle it poorly. So you should check what max length the model you want to use was trained to handle
Like when under Arab spring the Egyptian politicians tried to get the military involved to stop the protests, and got back (paraphrased)
“Our primary job is to protect the Egyptian people from violence. You really don’t want us involved in this”
If I go to a restaurant and order risotto, I haven’t made the dish, I’ve only consumed it. I want you to focus on that word “consume”, it’s important here.
If I buy a bread at the bakery, ham and cheese in the grocery store, and make me a sandwich, who’s the creator?
Hmm… what about pendulum painting? Where you put paint in a bucket, put a hole in it, and let it swing back and forth over the canvas?
On one side he chooses paint and size of hole and initial path and so on, but on the other hand he let nature and physics do the actual painting for him.
AI can be art. And you’re like the people criticizing the first photographers saying what they did wasn’t art. This is what I think.
And it’s going to have to be okay.
What do you think is “weight”?
You can call that confidence if you want, but it got very little to do with how “sure” the model is.
It just has to stop the process if the statistics don’t not provide enough to continue with confidence. If the data is all over the place and you have several “The capital of France is Berlin/Madrid/Milan”, it’s measurable compared to all data saying it is Paris. Not need for any kind of “understanding” of the meaning of the individual words, just measuring confidence on what next word should be.
Actually, it would be "The confidence of token Th is 0.95, the confidence of S is 0.32, the confidence of … " and so on for each possible token, many llm’s have around 16k-32k token vocabulary. Most will be at or near 0. So you pick Th, and then token “e” will probably be very high next, then a space token, then… Anyway, the confidence of the word “Paris” won’t be until far into the generation.
Now there is some overseeing logic in a way, if you ask what the capitol of a non existent country is it’ll say there’s no such country, but is that because it understands it doesn’t know, or the training data has enough examples of such that it has the statistical data for writing out such an answer?
IDK what did you do, but slm don’t really hallucinate that much, if at all.
I assume by SLM you mean smaller LLM’s like for example mistral 7b and llama3.1 8b? Well those were the kind of models I did try for local RAG.
Well, it was before llama3, but I remember trying mistral, mixtral, llama2 70b, command-r, phi, vicuna, yi, and a few others. They all made mistakes.
I especially remember one case where a product manual had this text : “If the same or a newer version of <product> is already installed on the computer, then the <product> installation will be aborted, and the currently installed version will be maintained” and the question was “What happens if an older version of <product> is already installed?” and every local model answered that then that version will be kept and the installation will be aborted.
When trying with OpenAI’s latest model at that time, I think 4, it got it right. In general, about 1 in ~5-7 answers to RAG backed questions were wrong, depending on the model and type of question. I could usually reword the question to get the correct answer, but to do that you kinda already have to know the answer is wrong. Which defeats the whole point of it.
You fucking imbecile. If women are locked in the bedroom how can they make dinner?? Moran